What is Generative AI?
Generative AI is a type of artificial intelligent model particularly designed for generating new. In contrast to conventional AI models, which mainly emphasize pattern identification or forecasting, generative AI generates new content informed by the data it has absorbed.
Key Features of Generative AI
- Imagination: It has the capability to create human-content.
- Adaptability: It gets better with time and experience.
- Automation: Minimizes human work in creative roles.
- Scalability: It can generate lots of content in a short time.
- Customization: It can personalize content to adapt it to individual needs and demand.
How Does Generative AI: Revolutionizing in ML Work?
Such models enable generative AI which is based on both these complex ML models, such as Generative Adversarial Network (GAN) and Transformer-based architectures( GPT). These models enable machines to generate original content by learning patterns, styles, and structures from vast datasets.
Here’s a breakdown of how generative AI works, step by step:
1. Data Collection
Generative AI begins with gathering and organizing large volumes of data.
- Types of Data: This could include text, images, videos, audio files, or numerical data.
- Purpose: The data serves as a foundation, helping the AI understand specific patterns and relationships.
- Example: An image-generating AI might be trained on millions of photographs to understand shapes, colors, and textures.
2. Training the Model
The collected data is fed into the AI model for training.
- Learning Patterns: The AI identifies patterns, relationships, and nuances within the data.
- Model Structures:
- GANs: These use two networks—a generator (creates content) and a discriminator (evaluates authenticity)—working together to improve results.
-
Transformers (e.g. GPT): This type experiments with understanding sequences and contexts through self-attention mechanisms, allowing AI to generate coherent text or perform tasks such as translation
- Output Development: The model learns to recreate or build on these patterns to produce realistic outputs.
3. Content Generation
Once trained, the model is ready to create new content.
- Input Prompts: Users provide prompts or queries, which guide the AI to generate specific outputs.
- Output Types: Depending on the model, outputs could include written articles, realistic images, audio compositions, or even 3D models.
- Example: Inputting “a cat sitting on a beach at sunset” into an image-generating AI produces a visual representation based on the description.
4. Fine-Tuning
After initial training, developers fine-tune the AI for better performance.
- Specialization: Adjustments are made to tailor the AI to specific tasks or industries, such as creating legal documents or designing virtual game worlds.
- Error Reduction: Developers refine outputs to minimize errors, biases, or inconsistencies.
- Continuous Updates: The AI is periodically updated with new data to keep it relevant and accurate.
Applications of Generative AI: Revolutionizing in ML
Generative AI is revolutionizing various industries. Below are some key applications:
1. Content Creation
- Writing blog posts, articles, and stories.
- Generating marketing copy and social media content.
2. Image and Video Generation
- Creating realistic images and animations.
- Generating deepfake videos for entertainment or educational purposes.
3. Music and Audio
- Composing original music tracks.
- Generating voiceovers or sound effects.
4. Healthcare
- Synthesizing medical images for research.
- Generating personalized treatment plans.
5. Gaming
- Creating realistic characters, environments, and storylines.
Generative AI Models: A Comparison
Model | Purpose | Examples | Strengths | Limitations |
---|---|---|---|---|
GANs | Image and video generation | StyleGAN, BigGAN | High-quality visuals | Requires extensive data |
GPT (Transformers) | Text generation | GPT-4, ChatGPT | Human-like text | Struggles with contextual depth |
DALL·E | Text-to-image conversion | DALL·E 2 | Creative, unique imagery | Limited editing capabilities |
Music AI | Audio and music composition | AIVA, OpenAI’s Jukebox | Original compositions | Limited genre understanding |
Benefits of Generative AI
Generative AI offers numerous advantages:
- Efficiency: Automates repetitive tasks.
- Creativity Boost: Provides inspiration and fresh ideas.
- Personalization: Tailors content to individual needs.
- Cost Reduction: Reduces reliance on human resources for creative tasks.
Challenges of Generative AI: Revolutionizing in ML in the Modern World
Despite its numerous benefits, generative AI faces several challenges that need to be addressed for its responsible use and continued growth:
1.Ethics — Potential for Misuse, Like Deepfakes or Mishav News
While generative AI may have the potential to be used for nefarious things, its ascendance has given us pause. For example, AI models can create very realistic but completely fictional content, like a deepfake video or a fake news article. This threatens public trust, privacy and security.
2.Post Processing: Making Sure the Output Fulfills Maximum To Be Reduced
Generative AI can generate impressive content but maintaining the quality of that content can be challenging. Often encounters things like misinformation, or even errors and inconsistencies, and lack the creative ways to handle it that a person does.
3.Model Training Requires a Lot of Computational Resources
Generating models of AI is an energy and compute-consuming process. It drives up costs and environmental damage. Moreover, such technology can remain out of reach to smaller organizations due to high cost of hardware and specialized infrastructure.
How to Get Started with Generative AI?
If you’re new to generative AI, follow these steps:
- Learn the Basics:
-
Fundamentals There was a good amount of knowledge that I needed to catch up on.
-
- Choose a Platform:
-
User-friendly tools are available from OpenAI, Hugging Face and RunwayML.
-
- Experiment:
-
Train on models pre-trained on text, generate the content, keep honing your skills.
-
- Join Communities:
-
Experimenting with these innovative tools will help you advance along the knowledge curve.
-
FAQs on Generative AI: Revolutionizing in ML
1.Can generative AI replicate human creative thinking?
No, it will bolster creativity by taking care of repetitive tasks and offering inspiration, but it cannot duplicate human creativity.
2. Is generative AI ethical?
It depends on how it’s used.Responsible practices will help mitigate risks — like misuse or bias.
3.Do I have to have coding skills to be able to use generative AI?
Not always. There are lots of platforms with no-code tools.
4. What are some free resources to learn about generative AI?
- OpenAI’s free demos.
- Coursera and Khan Academy online courses
The Future of Generative AI: Revolutionizing in ML
Here is just a look at what this cutting-edge technology has coming next as research and development advances:
1. Improved Accuracy: Smarter and More Context-Aware Models(Generative AI: Revolutionizing in ML)
Future generative AI models will become more precise and reliable.
- Deeper Context Understanding: AI will better grasp subtleties, such as emotional tones in writing or cultural nuances in visuals.
- Fewer Errors: Models will reduce inaccuracies, such as factual mistakes in text generation or artifacts in image creation.
- Personalization: AI could generate highly tailored outputs, catering to specific user preferences or industries.
- Example: Imagine an AI that crafts a story not only based on your prompt but also your unique writing style.
2. Broader Applications: Expanding Across New Domains
Generative AI will probably have all sorts of new applications across a continually expanding range of domains.
- Education: AI can build personalized lesson plans, engages with students through interactive study aids or virtual tutors.
- Healthcare: Simulating patient cases, generating synthetic medical data for research, or aiding in drug discovery.
- Agriculture: So this might be optimizing when to plant crops, modeling in 3D the patterns of how crops grow or creating new agricultural technologies.(Generative AI: Revolutionizing in ML)
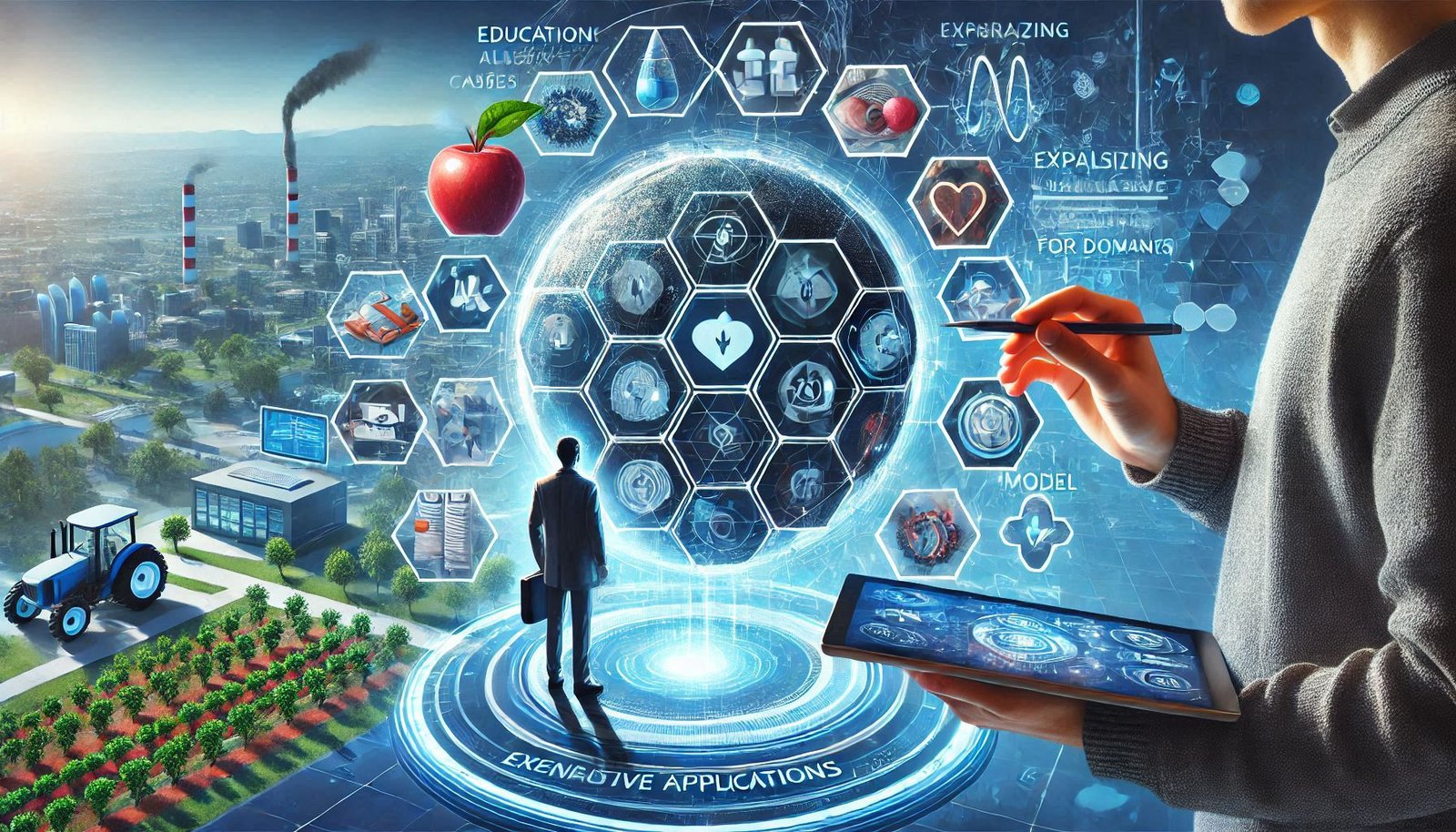
4 thoughts on “Generative AI: Revolutionizing in ML”